Abstract
Artificial intelligence (AI)-assisted robotic surgery seems to offer promise for improving patients’ outcomes and innovating surgical care. This commentary on a hypothetical case considers ethical questions that AI-facilitated surgical robotics pose for patient safety, patient autonomy, confidentiality and privacy, informed consent, and surgical training. This commentary also offers strategies for mitigating risk in surgical innovation.
Case
Ms A is a 50-year-old woman with a history of right breast cancer that was treated with mastectomy, axillary lymph node dissection, and radiotherapy and was complicated by severe lymphedema not amenable to nonoperative therapy. Ms A’s surgical history includes a laparoscopic appendectomy and 2 cesarean sections; her BMI is 32, and she is generally in good health.
Ms A has no clinical background but has researched surgical lymphedema therapy. She has spoken with patients who have undergone traditional surgical management of their lymphedema with vascularized omental lymphatic transplant using an open approach. A conventional open approach involves a longitudinal laparotomy incision from above the umbilicus to the xiphoid. This is more invasive than laparoscopic or robotic techniques as it requires a large incision and exposure, which carry increased risks of wound healing complications, surgical site infection, and less optimal scar aesthetics. In Ms A’s case, the surgeon, Dr B, recommends a minimally invasive, artificial intelligence (AI)-assisted robotic approach for omental harvest. Suppose the robotic platform is currently US Food and Drug Administration (FDA)-approved for urologic indications. Dr B indicates that emerging data about an AI-assisted approach are favorable but that research will be advanced by collecting data during Ms A’s operation.
Ms A wants surgical intervention for her lymphedema, as it has worsened despite over 6 months of nonsurgical management, and she is apprehensive about undergoing a new, clinically untested procedure. In particular, she worries that even though Dr B will be in the operating room during the entire case, an automated machine will be performing her surgery at certain points. Ms A also wonders which data will be collected and how her data will be stored, secured, and applied in the future.
Commentary
Ms A’s case demonstrates the ethical considerations attendant on the development of AI and robotic surgery. AI most simply refers to “the science and engineering of making intelligent machines, especially intelligent computer programs” to mimic the decision-making and problem-solving capabilities of the human mind.1 Machine learning is a subfield within AI that trains algorithms on data to gradually improve their accuracy in a manner that imitates how humans learn.2 While machine learning has become more commonplace in the public and military sectors, its role in health care remains under scrutiny.3,4,5,6,7 Biases are known to be incorporated in AI programs, which could perpetuate social inequality and harm patients.8,9 However, AI-assisted technology has the potential to greatly mitigate the global burden of disease by improving access to necessary medical and surgical care. Most AI-assisted technology has been utilized in preoperative planning and intraoperative guidance.10 Currently, autonomous surgical technology is in its preliminary stages of use in the operating room and in clinical trials in the areas of urologic, gynecologic, spine, and gastroenterological procedures.11,12,13 Could AI-assisted technology safely and ethically replace humans in the surgical arena? Indeed, it is conceivable that robots will be able to perform surgery relatively independently, with minimal assistance, although there is disagreement about the desirability and attainability of this goal.10,14,15,16 This paper will highlight potential issues and implications of this path.
Guiding Ethical Questions
Several key ethical issues must be considered in implementing AI-assisted technology in surgery (see Table).3,17,18,19
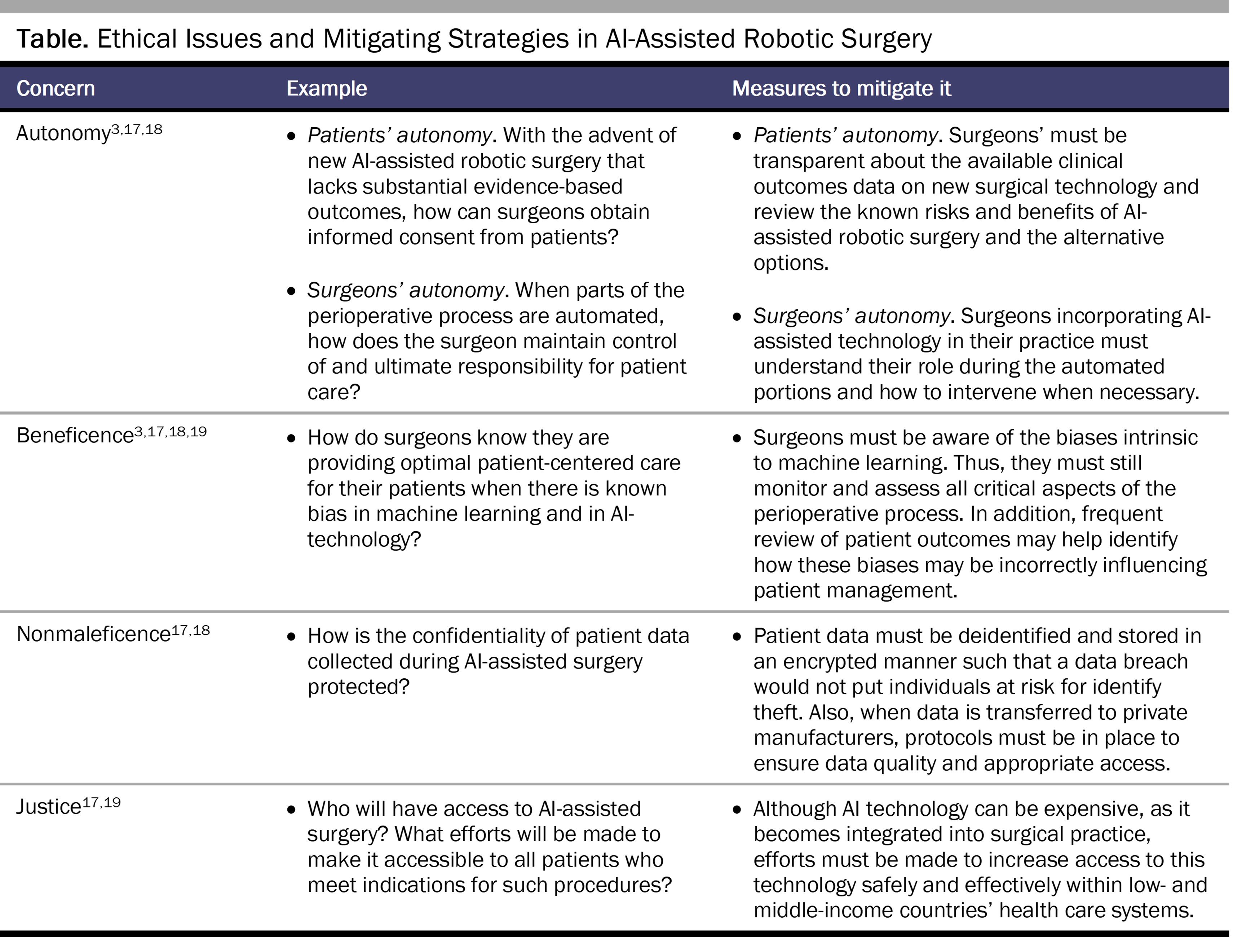
Patient autonomy and informed consent. How can patient autonomy be respected and informed consent assured, particularly given that the surgeon is using new technology without evidence-based results? Informed consent is critical to patient-centered care that respects patient autonomy and upholds the principles of beneficence and nonmaleficence. General components of informed consent include disclosing the risks and benefits of the procedure as well as alternative treatment options.20,21 In addition, the patient (or guardian) must demonstrate a reasonable understanding of the potential implications of the medical procedures to which consent is given. In this case, the surgeon must clearly explain what is known regarding AI-assisted robotic omental harvest and what remains unknown and discuss alternative options, such as robotic-assisted omental harvest (without AI support) or an open approach.
Ideally, a clinician will recognize when a patient is apprehensive, such as in this case, and ensure that all relevant information—including that which might dissuade the patient from providing consent—is disclosed. Of note, because the decision-making process of machine learning algorithms is a “black box” even to the programmers, the surgeon offering the AI-assisted surgery can’t possibly know exactly how the technology works, and this lack of knowledge must also be disclosed during the informed consent process. Finally, in this case, because the new (hypothetical) procedure has not yet been proven safe based on extensive clinical experience, obtaining true informed consent might not be possible. For non-FDA approved AI technology, potentially internal review board approval for each case (or case series) or a unique disclosure on surgical consents should be required to ensure that the surgeon appropriately discusses with the patient the novelty of the AI-assisted technology used in a specific procedure.
Suppose there is evidence that AI-assisted robotic procedures have better outcomes than the prior standard of care. How should a surgeon navigate a situation in which a patient still refuses to have AI-assisted robotic surgery while respecting patient autonomy? Surgeons are responsible for making clinical decisions that, in general, are in the best interests of patients so long as they do not violate patients’ autonomy. This process involves offering and ultimately recommending therapeutic options that are most likely to result in an optimal clinical outcome and that align with a patient’s values and wishes. In the current situation, Dr B ought to fully explain to Ms A that AI-assisted robotic omental harvest will likely lead to a better outcome than the alternatives based on available data and reported experience. However, if Ms A understands the likely outcome of each option yet still wishes to undergo the previous standard of care treatment, then Dr B should honor her autonomy and perform the standard procedure. If Dr B is not technically comfortable performing such a procedure, appropriate consultation should be sought, which might include recommending that the patient see a different surgeon with more experience in the preferred procedure.
Identifying and minimizing bias in AI-assisted surgery. Given each patient’s unique medical and surgical history, anatomy, and other features, how can we ensure that AI-assisted technology facilitates patient-centered and individualized care (ie, during an automated portion of a procedure)? Even though machine learning algorithms train on vast amounts of data to enable accurate diagnoses and prognoses and delivery of more equitable care, bias in AI has been well documented in the business, criminal, and health care literature.22,23,24,25,26 For example, machine learning algorithms likely will incorrectly estimate risks of certain diseases in patient populations that tend to have missing data in the electronic health record,8 with deleterious consequences. To take another example, in a study of machine learning algorithms for predicting intensive care unit mortality, algorithmic bias was shown with respect to gender and insurance type.27 This finding suggests that bias in training data for machine learning could lead to bias in algorithms, which then might falsely predict the risk of a disease (eg, breast cancer) in a specific population (eg, Black patients).
Additionally, how data are collected can introduce bias in training data. For example, collecting relatively more data from neighborhoods with higher police presence can result in more recorded crimes, which perpetrate more policing.28 If such unrepresentative data are used in training sets, the AI model will be biased.29,30 Thus, relying on AI during automated surgical care carries the risk of bias, with the potential to inadvertently harm the patient. However, the surgeon must acknowledge that human decision making is also affected by unconscious personal and societal biases and can be flawed.26 Whether AI decisions are less biased than human ones has not yet been proven.29
Before safely implementing AI in surgical settings, the risk of discrimination must be disclosed to a patient and potential harms discussed. It is also imperative that procedures for which AI-assisted technology functions independently of the surgeon be thoroughly evaluated before being applied in clinical practice. They might require human monitoring or supervision to ensure patient safety. Such monitoring during relevant portions of a procedure might reduce potential risks to the patient that could result from AI-assisted bias. For example, if there is an acute change in vitals or certain blood chemistry levels during surgery, an AI algorithm for such situations might not be as reliable as human judgment for that specific patient. Accordingly, the surgeon must explain in appropriate detail to the patient when the automated parts of the procedure occur and what her role is during that period. Optimal intraoperative decision making involves integrating patient information, evidence-based information, and surgical experience. To date, no AI-assisted surgical technology exists that achieves this goal, nor has any such technology been tested extensively with reproducible results in a large human patient cohort.28,31 Thus, human supervision and input during surgical procedures that use AI technology are necessary for the foreseeable future.
Nonmaleficence in data collection. How are data that are collected intraoperatively stored, and who owns and controls the data? How can we safeguard patient confidentiality in the automated world? If there is a data breach, what are the potential harms to patients? It should first be noted that it remains unclear in many cases who the owner of the data is; every state has different laws regarding medical record ownership.32 This question could be answered by future litigation and case law. Nevertheless, while electronic medical records and the increasing use of AI-assisted technology in health care have led to the growth of large digital medical databases that have the advantages of facilitated access, distribution, and mobility, there is a greater risk of a data breach.33,34 If patient medical data is breached, the potential harms to patients include psycho-emotional stress and identity theft, which can lead to false medical bills and the potential for unreliable medical records and subsequent life-threatening errors in medical decision making.35,36
To date, data collected intraoperatively (such as patient demographics, lab values, and outcomes such as specific morbidities and mortalities) are generally stored and managed by private AI health companies. These data are highly sought after to build AI algorithms for medical practice, not just for perioperative needs. Methods to protect patients from data breaches necessitate that AI health companies abide by federal and state laws and regulations regarding patient medical data. To abide by the Health Insurance Portability and Accountability Act (HIPAA),37 entities covered by HIPAA regulations, such as health care organizations, must deidentify personal health information before it can be stored on an AI health company database. Once deidentified, the clinical data are privately owned by an AI health company (eg, Google’s DeepMindTM, QuidTM, INFORMAITM, or BioSymetrics). Continued efforts by the AI health company to maintain privacy and protection of the data, as well as to properly train their employees in HIPAA compliance, are also paramount.35 Finally, if a data breach occurs, the patient must be informed by their clinician or the AI health company storing the data.
AI Technology and Roles of Surgeons
As the field of surgery evolves, there is a movement away from more invasive, human-influenced to minimally invasive, more machine-automated procedures.38 Some argue that the main tasks of surgeons are shared clinical decision making and performing operations, and both tasks have human limitations.38 A recent observational study demonstrated that cognitive error in the execution of care was the most common human performance deficiency associated with adverse surgical events.39 Thus, many supporters of AI-assisted technology believe that it could overcome human limitations and improve health care delivery. However, during this early transition period, as AI is incorporated in mainstream health care, the surgeon-in-training faces the reality that traditional surgeon-centered, surgeon-dependent procedures might become a thing of the past. Surgeons’ role could be more one of “computer operator” than “human operator.” But this change will be gradual over a long period.
In addition, during this transition period, mid-career surgeons who are very adept at current surgical techniques are faced with learning something new and essentially starting from the beginning of training. While any new surgical technique is being integrated, there is the risk of compromising results, but this risk can be mitigated by a surgeon’s careful practice, training, and mentorship by another surgeon more practiced in the new technique. Once that learning curve has been overcome, the surgeon can safely offer this new technique to their patients. Similarly, if a surgeon is more comfortable with the AI-assisted robotic surgery and not with the traditional open approach, the optimal safety plan would be to have another surgeon available to assist if a situation occurred in which the surgery needed to be converted to an open approach. Thus, careful planning would need to be done prior to a surgeon’s entering the operating room, as early adoption of technology does bring risks of user errors. For example, due to deaths occurring during robotic heart surgery, some surgeons are adamantly arguing for only human-controlled open-heart surgery.40 New technology is flashy and attractive for advertising purposes. However, to promote Aristotelian ethics and an emphasis on virtuous character and conduct, surgeons must assess and incorporate AI-assisted surgical technology with healthy skepticism.
Conclusion
Emerging AI technology in surgical care has many potential benefits, particularly in increasing access to and availability of necessary surgical care. However, this technology has known risks of bias and data breach, and the simple fact is that humans might never fully understand machine learning. As Ralph Waldo Emerson wrote in Self Reliance, “the civilized man has built a coach, but has lost the use of his feet”41; for junior surgeons in training, it is essential to continue to learn manual, surgeon-dependent skills while paying attention to evolving AI-assisted technology14,15,16,42,43 and considering the adoption of such technology in practice if it might improve patient care. However, the value of human clinical judgment, compassion, and flexibility in patient-centered care should not—and is unlikely to—be trumped by efficient, intelligent machines.
References
-
McCarthy J. What is AI? Basic questions. Accessed February 6, 2023. http://jmc.stanford.edu/artificial-intelligence/what-is-ai/index.html#:~:text=What%20is%20artificial%20intelligence%3F,methods%20that%20are%20biologically%20observable
-
Samuel AL. Some studies in machine learning using the game of checkers. II—recent progress. In: Levy DNL, ed. Computer Games I. Springer;1988:366-400.
-
O’Sullivan S, Nevejans N, Allen C, et al. Legal, regulatory, and ethical frameworks for development of standards in artificial intelligence (AI) and autonomous robotic surgery. Int J Med Robot. 2019;15(1):e1968.
- Morris MX, Song EY, Rajesh A, Asaad M, Phillips BT. Ethical, legal, and financial considerations of artificial intelligence in surgery. Am Surg. 2023;89(1):55-60.
-
Wangmo T, Lipps M, Kressig RW, Ienca M. Ethical concerns with the use of intelligent assistive technology: findings from a qualitative study with professional stakeholders. BMC Med Ethics. 2019;20(1):98.
-
Hung L, Mann J, Perry J, Berndt A, Wong J. Technological risks and ethical implications of using robots in long-term care. J Rehabil Assist Technol Eng. 2022;9:20556683221106917.
-
Carter SM, Rogers W, Win KT, Frazer H, Richards B, Houssami N. The ethical, legal and social implications of using artificial intelligence systems in breast cancer care. Breast. 2020;49:25-32.
- Parikh RB, Teeple S, Navathe AS. Addressing bias in artificial intelligence in health care. JAMA. 2019;322(24):2377-2378.
- Nelson GS. Bias in artificial intelligence. N C Med J. 2019;80(4):220-222.
- Zhou XY, Guo Y, Shen M, Yang GZ. Application of artificial intelligence in surgery. Front Med. 2020;14(4):417-430.
- Andras I, Mazzone E, van Leeuwen FWB, et al. Artificial intelligence and robotics: a combination that is changing the operating room. World J Urol. 2020;38(10):2359-2366.
- Okagawa Y, Abe S, Yamada M, Oda I, Saito Y. Artificial intelligence in endoscopy. Dig Dis Sci. 2022;67(5):1553-1572.
- Rasouli JJ, Shao J, Neifert S, et al. Artificial intelligence and robotics in spine surgery. Global Spine J. 2021;11(4):556-564.
- Bodenstedt S, Wagner M, Müller-Stich BP, Weitz J, Speidel S. Artificial intelligence-assisted surgery: potential and challenges. Visc Med. 2020;36(6):450-455.
-
Han J, Davids J, Ashrafian H, Darzi A, Elson DS, Sodergren M. A systematic review of robotic surgery: from supervised paradigms to fully autonomous robotic approaches. Int J Med Robot. 2022;18(2):e2358.
- Beyaz S. A brief history of artificial intelligence and robotic surgery in orthopedics & traumatology and future expectations. Jt Dis Relat Surg. 2020;31(3):653-655.
- Cobianchi L, Verde JM, Loftus TJ, et al. Artificial intelligence and surgery: ethical dilemmas and open issues. J Am Coll Surg. 2022;235(2):268-275.
-
Gerke S, Minssen T, Cohen G. Ethical and legal challenges of artificial intelligence-driven healthcare. In: Bohr A, Memarzadeh K, eds. Artificial Intelligence in Healthcare. Elsevier; 2020:295-336.
- Torain MJ, Maragh-Bass AC, Dankwa-Mullen I, et al. Surgical disparities: a comprehensive review and new conceptual framework. J Am Coll Surg. 2016;223(2):408-418.
- Reider AE, Dahlinghaus AB. The impact of new technology on informed consent. Compr Ophthalmol Update. 2006;7(6):299-302.
- Moore HN, de Paula TR, Keller DS. Needs assessment for patient-centered education and outcome metrics in robotic surgery. Surg Endosc. 2023;37(5):3968-3973.
-
Ntoutsi E, Fafalios P, Gadiraju U, et al. Bias in data-driven artificial intelligence systems—an introductory survey. Wiley Interdiscip Rev Data Min Knowl Discov. 2020;10(3):e1356.
-
Huang Y. Racial bias in software. Lipstick Alley. July 4, 2020. Accessed May 1, 2023. https://www.lipstickalley.com/threads/racial-bias-in-software.4263325/
-
Gijsberts CM, Groenewegen KA, Hoefer IE, et al. Race/ethnic differences in the associations of the Framingham risk factors with carotid IMT and cardiovascular events. PLoS One. 2015;10(7):e0132321.
-
Angwin J, Larson J, Mattu S, Kirchner L. Machine bias risk assessments in criminal sentencing. ProPublica. May 23, 2016. Accessed April 28, 2023. https://www.propublica.org/article/machine-bias-risk-assessments-in-criminal-sentencing
- Myers RP, Shaheen AAM, Aspinall AI, Quinn RR, Burak KW. Gender, renal function, and outcomes on the liver transplant waiting list: assessment of revised MELD including estimated glomerular filtration rate. J Hepatol. 2011;54(3):462-470.
-
Chen IY, Szolovits P, Ghassemi M. Can AI help reduce disparities in general medical and mental health care? AMA J Ethics. 2019;21(2):E167-E179.
-
Lum K, Isaac W. To predict and serve? Significance. 2016;13(5):14-19.
-
Hao K. This is how AI bias really happens—and why it’s so hard to fix. MIT Technol Rev. February 4, 2019. Accessed April 28, 2023. https://www.technologyreview.com/2019/02/04/137602/this-is-how-ai-bias-really-happensand-why-its-so-hard-to-fix/
-
Silberg J, Manyika J. Notes from the AI frontier: tackling bias in AI (and in humans). McKinsey Global Institute; 2019. Accessed April 28, 2023. https://www.mckinsey.com/~/media/mckinsey/featured%20insights/artificial%20intelligence/tackling%20bias%20in%20artificial%20intelligence%20and%20in%20humans/mgi-tackling-bias-in-ai-june-2019.ashx
-
Muradore R, Fiorini P, Akgun G, et al. Development of a cognitive robotic system for simple surgical tasks. Int J Adv Robot Syst. 2015;12(4):37.
-
Sharma R. Who really owns your health data. Forbes. April 23, 2018. Accessed April 28, 2023. https://www.forbes.com/sites/forbestechcouncil/2018/04/23/who-really-owns-your-health-data/?sh=4b67ceaf6d62
-
Shademan A, Decker RS, Opfermann JD, Leonard S, Krieger A, Kim PC. Supervised autonomous robotic soft tissue surgery. Sci Transl Med. 2016;8(337):337ra364.
- Kamoun F, Nicho M. Human and organizational factors of healthcare data breaches: the swiss cheese model of data breach causation and prevention. Int J Healthc Inf Syst Inform. 2014;9(1):42-60.
-
Seh AH, Zarour M, Alenezi M, et al. Healthcare data breaches: insights and implications. Healthcare (Basel). 2020;8(2):133.
-
Solove DJ, Citron DK. Risk and anxiety: a theory of data-breach harms. Tex Law Rev. 2018;96:737-786.
-
Security and Privacy. 45 CFR pt 164 (2023).
-
Loftus TJ, Filiberto AC, Balch J, et al. Intelligent, autonomous machines in surgery. J Surg Res. 2020;253:92-99.
-
Suliburk JW, Buck QM, Pirko CJ, et al. Analysis of human performance deficiencies associated with surgical adverse events. JAMA Netw Open. 2019;2(7):e198067.
-
Donnelly L. Robotic surgery is causing deaths heart surgeon warns—as he says he would opt for a traditional procedure. Telegraph. November 9, 2018. Accessed March 7, 2023. https://www.telegraph.co.uk/news/2018/11/08/tougher-rules-demanded-robotic-surgery-catalogue-errors-leads/
-
Emerson RW. Self-reliance. Caxton Society; 1909.
-
Moglia A, Georgiou K, Georgiou E, Satava RM, Cuschieri A. A systematic review on artificial intelligence in robot-assisted surgery. Int J Surg. 2021;95:106151.
- Chang TC, Seufert C, Eminaga O, Shkolyar E, Hu JC, Liao JC. Current trends in artificial intelligence application for endourology and robotic surgery. Urol Clin North Am. 2021;48(1):151-160.